Leveraging ChatGPT as a PM: part I (designing A/B experiments)
Kicking off a series of posts on how one can leverage ChatGPT / GPT-4 to their advantage as a PM (or anyone working in tech, really)
As most of you are probably well aware by now, there has been a ton of hype around ChatGPT (and especially around GPT-4 that has now been made available as part of ChatGPT offering) lately, with reactions covering a very wide range, including people voicing opinions like:
We are now on the verge of achieving AGI (Artificial General Intelligence), and that’s good/bad/extremely dangerous (my opinion: achieving AGI with current tech is very unlikely)
ChatGPT & other LLMs (Large Language Models) are awesome, and we’ll soon see many aspects of the human experience completely redefined by it (my take on this: we probably will, although perhaps in a less dramatic fashion that some are predicting, at least in the short-term)
ChatGPT trustworthiness & thus by extension usefulness are very low & because of this, ChatGPT & GPT-4 can be dangerous (my take: while the point about trustworthiness isn’t untrue per se, it feels that this position is overly pessimistic & is missing the point regarding the advantages a well thought-through usage of these tools can bring)
To be fair, most people aren’t necessarily taking extreme positions one way or the other, and there is certainly a lot of acknowledgement of the amazing progress ChatGPT & other LLMs represent. Still, it’s interesting to observe these extreme reactions, because in a way, you can view those as an ultimate testament to the fact that these tools are blowing many of the previous expectations out of the water, and are causing understandable cognitive dissonance as a result.
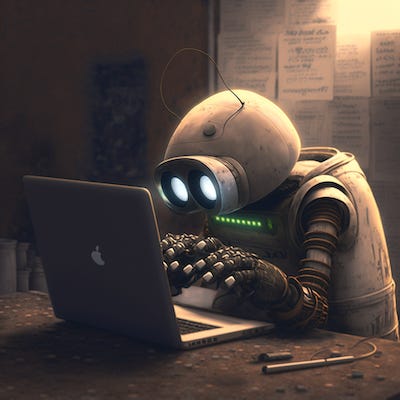
With this post (or, rather, what I’m hoping will become a series of posts), I intend to leave the esoteric debate about whether LLMs like GPT-4 represent an existential threat to humanity to all those already engaged in it (and it’s a very lively debate — if you don’t believe me, just check out Twitter these days!).
Instead, I’d like focus on describing my own (ongoing) experiments with GPT-4 & ChatGPT, in the effort to figure out the answer to a much more mundane question — namely, whether this tool can be put to a good use by the product managers (& perhaps others too) today, to help increase their productivity / unlock new use cases.
Please note that while my declared focus here is on Product Managers (since I’m one myself, and hence, this is what’s top of mind for me), in reality, a lot of things I am going to cover should be relevant to a lot of other people working in technology (& likely other industries too).
With that said, let’s dive in!
Today’s topic: Designing A/B experiments with the help of ChatGPT
Below, I’m going to share a series of prompts I submitted to ChatGPT, as well as ChatGPT’s responses (some edited for brevity). Then, I’m going to discuss the significance of this example as I see it, as well as the reasons why I think this type of stuff can be extremely useful to PMs (and a lot of other folks too).
Note that with these prompts, I was trying to emulate the PoV of a relatively inexperienced PM who wanted to run an A/B experiment, and was trying to learn more about the right way to set it up, and also how to analyze the results & explain them to others. This example is obviously a simplification of any real-world scenario, but I believe in many ways, it’s a very good illustration of what ChatGPT can do for you as a PM.
Me: Imagine we are running an A/B experiment trying to increase user engagement. We have around 10,000 users per month on average, using our product for 15 minutes a day. What would be the minimum detectable increase in engagement, assuming we want to get an 0.05 stat sig level from our experiment?
ChatGPT: To calculate the minimum detectable effect (MDE) in an A/B experiment, you need to provide the desired statistical power (e.g., 0.8 is a common choice), among other things. Also, you need to determine the proportion of users assigned to each group (e.g., 50% to the control group and 50% to the treatment group).
Me: What’s the definition of statistical power?
ChatGPT: Power is often denoted by the symbol "1 - β", where β is the probability of a Type II error, which occurs when the null hypothesis is not rejected even though the alternative hypothesis is true. A higher statistical power means a lower chance of committing a Type II error.
In most research settings, a power of 0.8 (or 80%) is considered adequate. This means that there is an 80% chance of detecting a true effect or difference if one exists. A higher power, such as 0.9, would provide even more confidence in the results but might require a larger sample size or a stronger effect to achieve.
Me: What's the definition of Type I & Type II errors?
ChatGPT: Type I and Type II errors are two types of errors that can occur in hypothesis testing.
A Type I error occurs when the null hypothesis is rejected even though it is actually true. This means that the researcher concludes there is an effect or difference when there is not one. The probability of making a Type I error is denoted by the symbol "α" and is typically set at 0.05 or 0.01.
A Type II error occurs when the null hypothesis is not rejected even though it is actually false. This means that the researcher fails to detect an effect or difference when one exists. The probability of making a Type II error is denoted by the symbol "β" and depends on the sample size, effect size, and statistical power.
In hypothesis testing, the goal is to minimize both Type I and Type II errors. However, reducing one type of error often increases the other type. The balance between the two types of errors is determined by the statistical power, sample size, effect size, and significance level.
Me: So, type I error is essentially false positive, and type II error is false negative, right?
ChatGPT: Yes, that's correct. A Type I error is equivalent to a false positive, meaning that the researcher concludes there is an effect or difference when there is not one. A Type II error, on the other hand, is equivalent to a false negative, meaning that the researcher fails to detect an effect or difference when one exists.
Me: So, with power of 0.8 & statistical significance of 0.05, I'll be making Type I error in 5% of cases & Type 2 error in 20% of cases on average, correct?
ChatGPT: [some back-and-forth (it initially misinterpreted what I said, and said ‘No’, but after some clarifications, it eventually arrived at the ‘Yes’ answer].
Me: Ok. Now assume I want to go for 0.01 significance level with the same sample size. How does it change power level calc?
ChatGPT: If you want to decrease the significance level from 0.05 to 0.01 while keeping the same sample size and effect size, it will result in a decrease in the power of the test. This is because a lower significance level means that the researcher is setting a higher bar for rejecting the null hypothesis, which requires a larger effect size or a larger sample size to achieve the same level of power.
To calculate the new power level, you would need to perform a power analysis based on the revised significance level and other relevant parameters such as the effect size and sample size. However, as a general rule of thumb, reducing the significance level from 0.05 to 0.01 may decrease the power of the test from 0.8 to around 0.6 or 0.7, assuming all other things are held constant.
Therefore, when considering changing the significance level, it is important to carefully consider the trade-offs between the risk of making Type I and Type II errors and the available resources, such as the sample size and effect size.
Side note: everything above was generated by GPT-3.5 (rather than the latest GPT-4). The reason for this is rather mundane — I simply ran out of ‘credits’ to use GPT-4 when I was experimenting with these prompts (even with a subscription, GPT-4 today is still being severely throttled, to accommodate all that demand, so you can only send it around 25 prompts every 3-4 hours).
Up until this point, GPT-3.5 did a good job though, so I didn’t feel the need to switch to GPT-4. However, for the subsequent prompts, where I asked it to generate me some numbers, GPT-3.5 surprisingly struggled, making undeclared assumptions & performing incorrect calculations, despite listing the right formulas (this part in particular was very surprising to me).
So, I decided to go back to GPT-4 & test if it can do a better job. It did, so all the prompts you see below are from GPT-4.
This also highlights certain limitations of ChatGPT today one has to be aware of, that I am going to discuss at the bottom of this post, once we are done with this example.
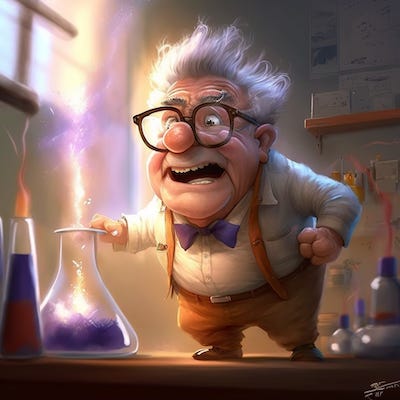
Me: Ok, now let's go back to the original question.
Assume we are running an A/B experiment trying to increase user engagement. We have around 10,000 users per month on average, using our product for 15 minutes a day on average. What would be the minimum detectable increase in engagement, assuming we want 0.05 stat sig level? Assume the split of 50/50 between treatment & control, and 0.8 power level.
ChatGPT: Let's calculate the minimum detectable effect (MDE) for your A/B experiment using the provided information:
10,000 users per month on average
15 minutes of usage per day on average
0.05 statistical significance level (alpha)
0.8 power level
50/50 split between treatment and control groups
Since the standard deviation (SD) was not provided, I'll provide MDE for a range of standard deviations (1, 2, 3, 4, and 5 minutes).
Determine t_alpha/2 and t_beta:
t_alpha/2 = 1.96 (for a two-tailed test with alpha = 0.05)
t_beta = 0.84 (for a power level of 0.8)
Calculate the required sample size (n) for each group:
Since there are 10,000 users per month and a 50/50 split between treatment and control, there will be 5,000 users in each group
Re-arrange the formula to solve for MDE and plug in the values for different standard deviations (SD): MDE = sqrt((t_alpha/2 + t_beta)^2 * (SD^2) / n)
SD = 1 minute: MDE = sqrt((1.96 + 0.84)^2 * (1^2) / 5000) MDE ≈ 0.062
SD = 2 minutes: MDE = sqrt((1.96 + 0.84)^2 * (2^2) / 5000) MDE ≈ 0.125
SD = 3 minutes: MDE = sqrt((1.96 + 0.84)^2 * (3^2) / 5000) MDE ≈ 0.187
SD = 4 minutes: MDE = sqrt((1.96 + 0.84)^2 * (4^2) / 5000) MDE ≈ 0.250
SD = 5 minutes: MDE = sqrt((1.96 + 0.84)^2 * (5^2) / 5000) MDE ≈ 0.312
Here are the minimum detectable effects for different standard deviations:
1-minute SD: MDE ≈ 0.062 minutes per day
2-minute SD: MDE ≈ 0.125 minutes per day
3-minute SD: MDE ≈ 0.187 minutes per day
4-minute SD: MDE ≈ 0.250 minutes per day
5-minute SD: MDE ≈ 0.312 minutes per day
These values represent the minimum detectable increase in engagement for each specified standard deviation, given a 0.05 statistical significance level and a 0.8 power level.
Me: But isn't the duration of the experiment supposed to play a role here as well? (e.g., whether we run it for 1 day, vs. 1 week vs. 1 month)
ChatGPT: You are correct; the duration of the experiment plays a role in determining the minimum detectable effect.
In my previous calculations, I did not account for the duration of the experiment. To account for the duration of the experiment, we need to adjust the sample size (n) to represent the number of user-days in each group rather than just the number of users.
Assuming you have 5,000 users in each group and you run the experiment for a certain number of days (d), the adjusted sample size (n') is: n' = n * d .
Now we can use the adjusted sample size to calculate the MDE:
MDE = sqrt((t_alpha/2 + t_beta)^2 * (SD^2) / n')
Let's consider three different experiment durations: 1 day, 1 week (7 days), and 1 month (30 days).
Experiment duration: 1 day:
n' = 5000 * 1 n' = 5000 ,
MDE = sqrt((1.96 + 0.84)^2 * (1^2) / 5000) ,
MDE ≈ 0.062
Experiment duration: 1 week (7 days):
n' = 5000 * 7 n' = 35000 ,
MDE = sqrt((1.96 + 0.84)^2 * (1^2) / 35000) ,
MDE ≈ 0.023
Experiment duration: 1 month (30 days):
n' = 5000 * 30 n' = 150000 ,
MDE = sqrt((1.96 + 0.84)^2 * (1^2) / 150000) ,
MDE ≈ 0.010
Here are the minimum detectable effects for different experiment durations:
1 day: MDE ≈ 0.062 minutes per day
1 week: MDE ≈ 0.023 minutes per day
1 month: MDE ≈ 0.010 minutes per day
As the duration of the experiment increases, the MDE decreases, making it easier to detect smaller changes in user engagement. This is because the larger sample size (more user-days) provides more statistical power to identify smaller effects.
Why I believe the above is awesome
What are your first thoughts, seeing the series of prompts above & the answers that ChatGPT generated? Let me know in the comments section below!
At the first glance, I can imagine how the exchange above might not strike everyone as anything particularly special (beyond the fact that you can have an actual productive dialog with ChatGPT, which, of course, is incredible on its own).
Here are some of the reasons why I think it’s awesome though:
Understanding the intuition behind applied statistics isn’t easy; explaining it to others is even harder — as shown above, ChatGPT can be extremely helpful with both these tasks
To be more specific, you wouldn’t believe how many folks I encountered who didn’t understand the concept of the minimum detectable effect — including people who had to make business decisions based on the experiments’ results
That’s why being able to clearly articulate the point, especially to less technical folks, can truly go a looooong way
Beyond just communicating the details on the setup of your experiment to others, I am hoping the above clearly demonstrates the value of ChatGPT as a learning tool — you can ask it questions, dig deeper, refine the task, and so on
You can also get ChatGPT to perform fairly complex back-of-the-envelope calculations for you, which can be very helpful both for understanding the nuances of A/B experimentation better, and getting some talking points to share with others
Some important limitations of ChatGPT today
As I mentioned above when I was describing the prompts & ChatGPT answers, ChatGPT today certainly isn’t flawless.
In particular:
It’s prone to making assumptions without always clearly communicating what those are (this seemed to be particularly true for GPT-3.5, and a bit less of a problem with GPT-4)
ChatGPT can ‘hallucinate’ in certain instances, meaning it would make things up — and oftentimes, these things will sound quite plausible too
This problem is currently being actively discussed / researched, and is one of the key reasons people (correctly) question the trustworthiness of GPTs & other LLMs
As I discovered while running a series of prompts for this example, you cannot automatically trust ChatGPT calculations either, even when it has the right formulas — it can still make mistakes
Are those limitations significant? Well, yes & no.
The propensity of ChatGPT (& other LLMs) to hallucinate certainly poses challenges (e.g., integrating those models into search can be quite tricky, since the quality & trustworthiness of response matters a lot there).
At the same time, if you are using ChatGPT to explore a topic you’re generally familiar with (and thus can notice & call out suspect info, as well as check calculations, etc.), I feel ChatGPT can be extremely useful in a variety of ways:
It can be a powerful tool to help you refresh your memory on the topic & learn more about it
It can also be a great productivity-enhancing tool, allowing you to quickly prototype & explore things (example above certainly falls into this bucket)
Finally, it can help you find better ways to explain the nature of the problem, nuances around it & your results to others
Conclusion
As I mentioned before, this post is just the first one in a series of articles exploring how we can make use of ChatGPT & other LLMs in our daily lives / work.
It’s not often that we get to witness the emergence of this kind of revolutionary technology, and, as is often the case with such advances, the difference between embracing it early on (& thus enhancing your own productivity), vs. burying your head in the sand & pretending nothing has changed, will likely be pretty stark in the long run.
From my early experiments, I believe ChatGPT can be extremely useful for PMs & many others, so I plan to cover various scenarios it can be used for over time, and would love to hear about the ways you’re using it as well!
Hopefully, this was helpful! And, as always, if you have any feedback you’d like to share, let me know in the comments section below!